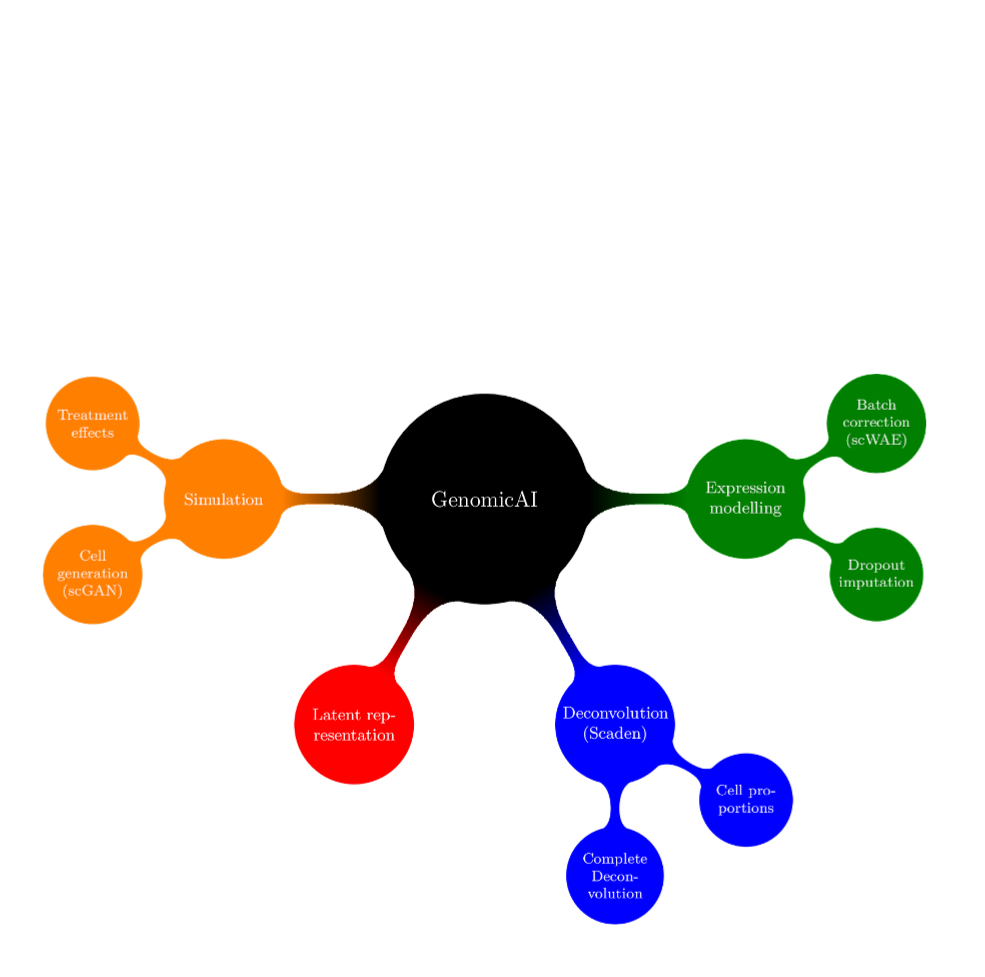
Biological systems are usually highly complex, as intracellular and intercellular communication, for example, are orchestrated via the non-linear interplay of tens to hundreds of thousands of different molecules. Recent technical advances have enabled scientists to scrutinize these complex interactions, measuring the expression of thousands of genes at the same time, for instance. Unfortunately, this complexity often becomes a major hurdle as the number of observations can be relatively small, due to economical or ethical considerations or simply because the number of available patient samples is low. Next to technically induced measurement biases, this problem of too few observations, in the face of many parameters, might be one of the most prominent bottlenecks in biomedical research. However, recent advances in Machine Learning have allowed to tackle those statistical challenges, making it possible to learn lower dimensional representations (e.g. latent representation of deep generative models) of genomic data (e.g. transcriptomics) that preserve the biological signal while removing or reducing noise or biases in the measurements. In turn, we use such representations to efficiently solve complex tasks such as batch effect correction, data augmentation, trajectory inference or imputation of dropout events in scRNA-seq data or deconvolution of the cell type composition in RNA-seq samples.
Members
Biological Sequences Data Analysis: developing TCR specificity prediction and Drug-Target interaction
single cell/nuclear RNA-seq analysis
Machine learning for scRNA-seq, Deconvolution
Alumni
Single-cell sequencing data analysis of immune cells in inflammatory diseases
Research on generative models for transcriptomic data to reconstruct missing expression information and other applications in the single-cell RNA-seq field
Stay In Touch